The Future of AI: Breaking Down Barriers with Machine Learning
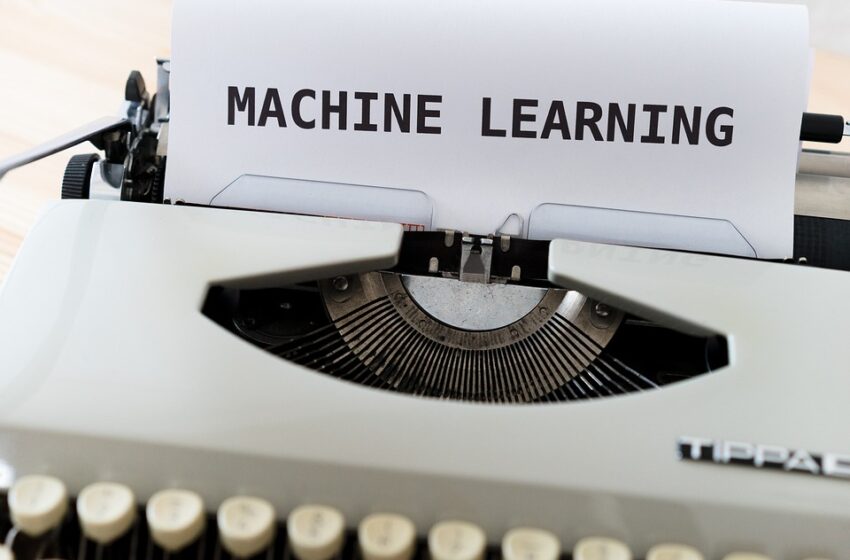
Artificial Intelligence (AI) has been a buzzword in technology for the past decade, with advancements in machine learning leading the way. Machine learning algorithms allow computers to learn from data and make predictions or decisions without being explicitly programmed. This technology has already revolutionized industries such as healthcare, finance, and transportation, and its potential applications are limitless.
One of the key barriers that AI faces is the lack of understanding and trust from the general public. Many people are concerned about the implications of AI on jobs, data privacy, and ethics. However, with advancements in machine learning, AI systems are becoming more transparent and interpretable, which is crucial for building trust with users.
Interpretability in machine learning refers to the ability to explain how a model arrived at a certain decision or prediction. This is important for ensuring that AI systems are fair, reliable, and free from bias. Researchers are developing techniques to make machine learning models more interpretable, such as feature importance analysis, model visualization, and rule extraction.
Another barrier to AI adoption is the lack of diverse and representative data. Machine learning models are only as good as the data they are trained on, and biased or incomplete data can lead to biased predictions. To break down this barrier, researchers are working on techniques to mitigate bias in data, such as data augmentation, synthetic data generation, and fairness-aware learning algorithms.
Furthermore, the computational power required to train and deploy machine learning models is a significant barrier for many organizations. Training deep learning models on large datasets can be computationally intensive and expensive. To address this challenge, researchers are developing techniques to optimize and accelerate the training process, such as distributed training, model pruning, and quantization.
As AI continues to advance, we can expect to see a convergence of machine learning with other technologies, such as robotics, natural language processing, and computer vision. This will enable AI systems to understand and interact with the world in more meaningful ways, leading to new applications in areas such as autonomous vehicles, personal assistants, and healthcare diagnostics.
In conclusion, the future of AI is bright, with machine learning playing a key role in breaking down barriers and unlocking the full potential of artificial intelligence. By addressing challenges such as interpretability, data bias, and computational complexity, we can build AI systems that are transparent, fair, and efficient, leading to a future where AI improves the lives of people around the world.